A Post Mortem on the Gino Case
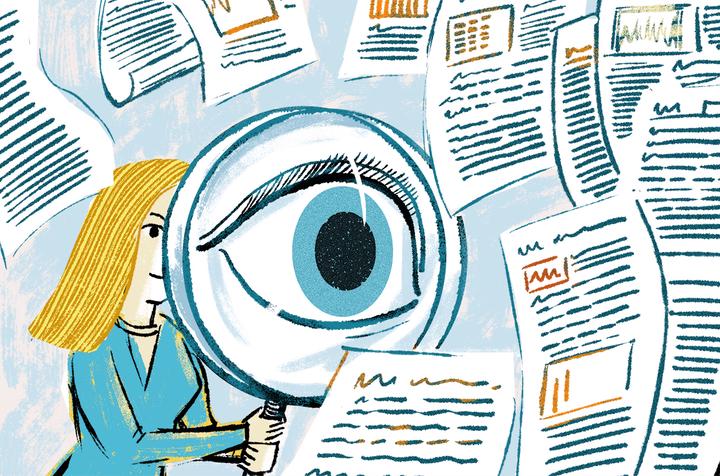
Disclaimer: None of the opinions expressed in this letter should be construed as statements of fact. They only reflect my experience with the research process, and my opinion regarding Francesca Gino’s work. I am also not claiming that Francesca Gino committed fraud: Only that there is overwhelming evidence of data fabrication in multiple papers for which she was responsible for the data.
On September 30th, 2023, the New Yorker published a long piece on “L’affaire Ariely/Gino”, and the role I played in it. I am grateful for the messages of support I received over the past few weeks. In this post, I wanted to share more about how I came to discover the anomalies in Francesca Gino’s work, and what I think we can learn from this unfortunate story.
What is The Story?
How it all began
I started having doubts about one of Francesca Gino’s paper (Casciaro, Gino, and Kouchaki, “The Contaminating Effect of Building Instrumental Ties: How Networking Can Make Us Feel Dirty”, ASQ, 2014; hereafter abbreviated as “CGK 2014” ) during my PhD. At the time, I was working on the topic of networking behaviors, and this paper is a cornerstone of the literature.
I formed the opinion that I shouldn’t use this paper as a building block in my research. Indeed, the idea that people would feel “physically dirty” when networking did not seem very plausible, and I knew that many results in Management and Psychology published around this time had been obtained through researchers' degrees of freedom. However, my advisor had a different view: The paper had been published in a top management journal by three prominent scholars… To her, it was inconceivable to simply disregard this paper.
I felt trapped: She kept insisting, for more than a year, that I had to build upon the paper… but I had serious doubts about the trustworthiness of the results. I didn’t suspect fraud: I simply thought that the results had been “cherry picked”. At the end of my third year into the program (i.e., in 2018), I finally decided to openly share with her my concerns about the paper. I also insisted that given how little we knew about networking discomfort, and given my doubts about the soundness of CGK 2014, it would be better to start from scratch and launch an exploratory study on the topic.
Her reaction was to vehemently dismiss my concerns, and to imply that I was making very serious accusations. I was stunned: Either she was unaware of the “replication crisis” in psychology (showing how easy it is to obtain false-positive results from questionable research practices), or she was aware of it but decided to ignore it. In both cases, it was a clear signal that it was time for me to distance myself from this supervisor.
I kept digging into the paper, and arrived at three conclusions:
The paper presents serious methodological and theoretical issues, the most severe being that it is based on a psychological mechanism (the “Macbeth Effect”) that has repeatedly failed to replicate.
The strength of evidence against the null presented in study 1 of the paper made it extremely unlikely that the result was p-hacked: It is statistically implausible to obtain such a low p-value under the null, even when using researchers' degrees of freedom.
Francesca Gino had many other papers that appeared equally implausible (i.e., untrustworthy psychological mechanisms leading to large effects with very low p-values).
It was at this point that I started suspecting that part of the evidence presented in CGK 2014 was not just p-hacked but based on fabricated data. At the time, I wasn’t clear how warranted these suspicions were, or about the best way to share them with anyone, as I did not have enough tangible elements to support or prove this suspicion.
What I knew, however, was that I had accumulated enough theoretical and empirical arguments to seriously question the conclusions of CGK 2014, and that these arguments might be of interest to the scientific community. Indeed, CGK 2014 is an unavoidable building block for anyone studying networking behavior: It is authored by influential scholars, published in a prestigious journal, received the Outstanding Publication Award in OB at the 2015 Academy of Management annual meeting for its “significant contribution to the advancement of the field of organizational behavior”. Sharing these concerns (again, independently of my suspicion of fraud) appeared like the right thing to do: It would bring awareness to the issues in CGK 2014, and hopefully spur novel investigations into the root causes of networking discomfort.
I therefore decided to write a 10-page criticism of the paper and to include it in the first chapter of my dissertation. This criticism summarized my argument against CGK 2014, explained why I chose not to rely on this paper in my research, and justified my choice of studying different psychological underpinnings of networking discomfort. This criticism, again, only focused on the theoretical and methodological issues in CGK 2014.
Shooting the messenger
The story so far is very banal. I, a (very) early-career researcher, took a deep dive into a famous paper and discovered inconsistencies. These stories always start with “that’s odd…”, “it doesn’t make any sense…”, or “there is something off here…”. Then, I second-guessed myself, a lot. After all, the authors are famous, serious people; and the paper is published in a prestigious peer-reviewed journal. So I thought “I must have misunderstood,” “I must be missing a part of the puzzle,” “it was probably addressed during the peer review process”… Then, as I finally grew more confident that the issues were real and substantial, I decided to write about them.
What should happen then (if science were, as many people like to say, “self-correcting”) is that, after a peer-review of some form, my criticism would get printed somewhere, and the field would welcome my analysis the same way it welcomes any other paper: Another brick in the wall of scientific knowledge.
As revealed in the New Yorker piece, this is not at all what happened. The three members of my committee (who oversaw the content of my dissertation) were very upset by this criticism. They never engaged with the content: Instead, they repeatedly suggested that a scientific criticism of a published paper had no place in a dissertation. After many frustrating exchanges, I decided to write a long letter explaining why I thought it was important to document the issues I had discovered in CGK 2014. This letter stressed that I was not criticizing the authors, only the article, and encouraged the members of my committee to highlight anything in my criticism that they viewed as inaccurate, insufficiently precise, or unfair.
The three committee members never replied to this letter. Given this lack of response, I decided to keep the criticism in the dissertation draft that was shared with them before my defense. On the day of the defense, external committee members called the criticism “unusual,” “unnecessary,” and argued that since I had not run a replication of the study, I could not criticize it. Only one committee member found it “brave and interesting.”
After the defense, two members of the committee made it clear they would not sign off on my dissertation until I removed all traces of my criticism of CGK 2014. Neither commented on the content of my criticism. Instead, one committee member implied that a criticism is fundamentally incompatible with the professional norms of academic research. She wrote that “academic research is a like a conversation at a cocktail party”, and that my criticism was akin to me “storming in and shouting ‘you suck’ when you should be saying ‘I hear where you’re coming from but have you considered X’”. The other committee member called my criticism “inflammatory,” and lambasted me for adopting what he called a “self-righteous posture” that was “not appropriate.”
At this point, the only option left for me was to cave. I was terrified that they would not allow me to graduate, disgusted to see such a blatant abuse of power, dismayed to think that all the work I had done documenting the issues in CGK 2014 would be in vain, and absolutely stunned that they did not view the issues I was raising as worth sharing. I ultimately submitted a “censored” version of the dissertation, determined to make the “director’s cut” publicly available online later.
From doubts to concerns
A few months later, I had an itch to run a replication of the key study (Study 1) of CGK 2014 and see if it would confirm my intuition that something was wrong. I contacted the three authors and asked them for the material and raw data. I obtained the material and a dataset (which was not the original Qualtrics data) from Gino. I also learned two things in my exchange with the authors:
- Casciaro and Kouchaki never had access to the data before the paper was published: They did not run the study, did not analyze the data, and did not have a copy of the data.
- Gino still had access to the Qualtrics survey (and therefore to the raw Qualtrics data) at the time I contacted her: The materials she sent me had just been printed from the Qualtrics survey.
I ran a replication of Study 1 of CGK 2014 using the authors' original materials. Not only did I fail to replicate the original result, but I also found serious anomalies when comparing the data of my replication to the data of the original. These anomalies confirmed my suspicion that there was probably something off with this data.
While collecting the materials for the replication of CGK 2014, I discovered that more data collected by Gino was available online. First, I found out that Casciaro, Gino and Kouchaki had published an extension of their 2014 paper (Gino, Kouchaki, and Casciaro, 2020) and made the data available on an OSF repository. I had seen an early version of this paper, and saw it contained the same patterns that concerned me in CGK 2014 (i.e., an implausibly large effect with a very small p-value). I also found that Gino had shared the data of another paper on the OSF (Gino, Kouchaki, and Galinsky, 2015), and that the data of the “signing at the top” paper (Shu, Mazar, Gino, Ariely, and Bazerman, 2012) had also been made available on the OSF following the failure to replicate the effect (Kristal, Whillans, Bazerman, Gino, Mazar, and Ariely, 2020).
I now had four datasets, and I needed help to analyze them. I therefore contacted another researcher who has chosen to stay anonymous. She and I worked together for several weeks on these four different datasets. Once we were finally convinced that we had found sufficient evidence of anomalies, we decided to reach out to Data Colada. They provided a fifth dataset they had access to, reran all our analyses, and discovered additional anomalous patterns.
Working with Data Colada
People seem to have a lot of incorrect ideas on how “data detectives” work, so I thought I would share a bit about my experience working with Data Colada.
The first thing that struck me was how incredibly cautious they have been throughout the entire process. They constantly played the devil’s advocate: Every single piece of evidence we brought them was met with skepticism, even the ones we thought were “smoking guns”. They were constantly looking for reasonable alternative explanations for the suspicious data patterns we were finding. Was it frustrating? It was! In my interactions with them, I sometimes felt that their standards of proof for fraud were simply impossible to meet. It was their way of being careful.
When looking at anomalies, Uri, Joe and Leif do not operate with a mindset of “what would convince us that the data is fabricated.” They instead judge evidence according to a much stricter standard: “What would convince almost anyone that the data is fabricated.” Indeed, they knew that if it turned out that several papers authored by Francesca Gino contained fabricated data, they would have to bring evidence to parties that are typically extremely reluctant to investigate. Anyone who has engaged in data forensics know that journals and institutions are strongly motivated to ignore evidence of fraud. Uri, Joe, and Leif knew that too, which is why they triple-checked everything and made sure that fabrication was the only reasonable explanation for the patterns we found.
Finally, I would like to finish this section by sharing a personal opinion about Uri, Joe and Leif. I have been extremely impressed by the decency, integrity, and patience with which they have handled the entire process, even more so since the publication of the first Data Falsificada post. Despite the innuendos, the lies, the lawsuit, and all the other stupid things that people have said about their work and their motives, they just keep doing what they’re good at: Doing science, revealing truth, teaching us to do better. If the average person in the field had their level of integrity and courage, I would probably not need to write these lines.
What Can We Learn from This Story?
Now that you know more about this story, I wanted to share some perspective on what I think we can (and should) learn from it. Again, these are my own thoughts, and I do not speak for Data Colada or for other whistleblowers.
From a truth-finding perspective, p-hacking is as damaging as fraud
P-hacking and fraud are treated very differently, and for good reasons. Fraud is much more deliberate and malicious, while p-hacking can (and will) happen anytime scholars do not pre-register their studies. However, the outcome for science is the same: Too many untrustworthy findings enter the literature.
In addition, p-hacked findings make fraud much harder to detect: It provides an alternative explanation to counter-intuitive, incredible, unreplicable findings. In a world in which ridiculous effects can be shown to “exist” thanks to p-hacking (i.e., showing that listening to music can make people younger), how does one identify fraudulent findings?
P-hacked effects also provide the implausible theoretical foundations on which fraudulent findings are built. Take the effect I failed to replicate: Networking makes people feel physically dirty. Ridiculous? Well, (p-hacked) research has shown that moral violations make people feel physically dirty, so if networking is a moral violation, isn’t it plausible that it makes people feel physically dirty?
Fraud (and p-hacking) have real consequences
Let’s talk about the human costs first. Think about all the people who try to replicate, extend, or build upon these false positives. These people are often graduate students, blissfully unaware of the fact that some effects are non-replicable. If, as a graduate student, you waste six months or a year working on a dead end, odds are you will never find a job in academia. By the time you realize you’ve been pouring resources down the drain, it’s already too late. You won’t have a publication by the end of your PhD, and most research-oriented universities will not consider you for an interview.
Let’s talk about opportunity costs then. Any resource spent trying to extend or replicate fake research is a resource that isn’t spent discovering real findings. Think of the amount of time and money that all the researchers in behavioral / social sciences have expended working on dead ends, and think how much more we would know about the human mind, the working of organizations, the behavior of individuals if we had done a better job keeping fraud and p-hacking at bay?
Let’s talk about crowding out effects finally. When a subset of scientists can reliably produce incredible effects (because they cut corners), and publish hundreds of papers, they set a bar that serious, careful researchers can never hope to meet.
Fraud and p-hacking generate huge negative externalities: Private gains lead to collective costs. While many (too many) have been able to build a career for themselves by publishing their p-hacked effects, our fields are more fragile and less credible than ever.
Fraud is too common (and too rewarding)
The incentives for fraud in business academia are significant. If you can meet the standards for hiring, promotion, and tenure at an R1 university (something that is much easier once you fabricate your data), you will get:
A 6-figure salary with full benefits until you retire
Complete job security
A flexible work environment (no boss, remote work…)
The social status and reputational benefits that go with the “Professor/Dr.” title
Opportunities to do book deals, TED talks, to teach in executive education, to conduct corporate workshops…
The benefits of fraud must be balanced with the risks of course. Are the risks of being caught for faking data high enough? I don’t think so:
The peer review process, as it exists today, makes it extremely difficult to catch fraud. Only a minority of journals require making data and materials available to reviewers, who are overworked and under-qualified to detect fraud. Even fewer journals require posting data and materials to the public. If fraud isn’t detected during peer-review, who will be able to catch it?
The bar to accuse someone of fraud is extremely high. Failing to replicate the effect? Not enough. Non-sensical effect sizes? Not enough. Anomalies in data? Not enough. Unless you can invest the resources to identify anomalous patterns of fraud across multiple papers, THEN drum up enough support from journals or universities to consider your suspicions, THEN hold their feet to the fire when they are unwilling to act… the probability that the person will never face consequences for fabricating data is very high.
The incentives to investigate and call out fraud are non-existent. In fact, the opposite is true: If you find something fishy in a paper, your mentor, colleagues, and friends will most likely suggest that you keep quiet and move on (or as I have learned the hard way, they might even try to bully you into silence). If you are crazy enough to ignore this advice, you are facing a Sisyphean task: Emailing authors to share data (which they do want not to), begging universities to investigate (which they do not want to), convincing journals to retract (which they do not want to), waiting months or years for them to share their findings with the public (if it ever happens)…
Business academia needs to reckon with this inconvenient truth: Committing fraud is, right now, a viable career strategy that can propel you at the top of the academic world. If fraud were extremely rare (as people who oppose scientific reforms like to say), what would be the odds that an investigation into Gino’s work would also reveal fraud in Ariely’s work? What would be the odds that, as reported in Gideon Lewis-Kraus' article, a re-analysis of Gino’s paper lead to the discovery of fraud committed by yet another person? How many dominoes need to fall before we make drastic changes to the system?
We need to make fraud harder to commit and easier to detect. It means having proper channels for people to report fraud, with solid guarantees that their identity will be protected and that they will face no repercussion. It means rewarding (not punishing) people who find and expose fraud. It means imposing harsher punishment on people who commit fraud. It means making data posting compulsory. It is unconscionable that in 2023, academics are still debating whether they need to share their data with the public. Data is an integral part of empirical papers: It is part of the scientific record, and researchers need to stop treating their data like family heirlooms.
Who will detect fraud and clean up the mess?
I have never worked on dishonesty. I did not read any of Gino’s papers on the topic prior to my suspicions that some of her data might have been fabricated. I stumbled upon her research only because she had written one paper on networking behaviors (which was my dissertation topic). As for the anonymous researcher who worked with me, she only discovered the anomaly in Ariely’s data because we were together looking at Francesca Gino’s research. In other words, we were some of the most unlikely persons to identify this fraud and raise red flags. Which raises the question: What were the parties most likely to find the fraud doing?
First, what about the dishonesty experts of the field? Did none of them have doubts about Gino’s or Ariely’s work? Not even after Gino claimed, in a meta-analysis on unethical behavior, that almost none of her data was available? Not even after the famous “sign at the top” paper failed to replicate, despite spectacularly strong effects in the original? Perhaps some of these experts were suspicious but stayed quiet… but if so, why? Were they pre-tenure researchers, afraid (for good reasons) that criticizing superstars of the field would doom their career? Were they post-tenure researchers who didn’t feel comfortable speaking out? Were they pressured or bullied into silence like I was? We need to confront these uncomfortable questions if we want to prevent future scandals.
Second, what about Gino’s co-authors and reviewers? While we might never know how many of her papers contained fabricated data, she has published 130+ papers over the course of 17 years, with approximately as many co-authors. Each of these papers was presumably assigned to two or three reviewers, one editor, presumably an associate editor too, for multiple rounds of reviews. What should we make of the fact that no one saw (or said) anything?
Some of Gino’s co-authors worked extensively with her. A dozen of them were regular collaborators, who wrote 5+ papers with her. Some of them are junior scholars, and are most likely under intense stress, dealing with the fallout of having a regular co-author suspected of data fabrication. We cannot fault them for staying quiet and dealing with these issues first. However, I am very disappointed with Gino’s more senior co-authors. It is shocking to me that, Juliana Schroeder excepted (who has sent a clear signal to the scientific community that she is taking this scandal seriously, and shared transparent guidelines on how she is evaluating the papers she has co-authored with Gino), none of these tenured co-authors have made any sort of public statement. Do they not think that the scientific community deserves more transparency? Do they not feel the need to signal whether and why people should still trust their work? I still have some hope that the repeatedly delayed Many Co-Authors project will shed some light on these questions… but I’m not holding my breath.
Finally, the journals and HBS deserve to be called out. The former for publishing Gino’s research, the latter for providing her with the resources that helped her become the superstar she was before her downfall. Now that it appears that some of Gino’s research was based on fabricated data, why aren’t they actively investigating all her papers? Why aren’t they helping the co-authors, and the rest of the scientific community, figure out the truth? After Diederik Stapel was terminated for research misconduct, Tilburg University conducted a comprehensive investigation into the entirety of his research output, and made the findings public. Why won’t HBS and the journals do the same thing? The silver lining is that HBS at least conducted an investigation and acted decisively, unlike Duke university after Data Colada [98] came out…
Research independence doesn’t mean lack of accountability
To do their work, researchers need freedom from political interventions, from the ebbs and flow of public opinion, or from the tight grip of a rigid hierarchy. However, this freedom doesn’t mean that researchers are accountable to no one. What should this accountability look like?
First, it means being as willing to take criticism as you are willing to take praise. All researchers are keen to accept awards, grants, or other professional accolades for the papers they publish. On the other hand, most researchers react defensively when people ask questions, require more details (e.g., data and materials), or even prove them wrong (e.g., by failing to replicate their effect, or pointing to a mistake or anomalies in the paper). If you do not want to deal with criticism, the best place for your manuscripts is in your drawer.
Second, researchers need to be more willing to criticize shoddy or unreliable research. Academic circles are tight-knit, such that researchers often express reluctance to criticize the research of someone who could be their next reviewer, their next colleague, the next person to hire their graduate students, or their next neighbor at a conference dinner’s table. This reluctance is, however, a complete abdication of the immense privilege of tenure. Tenure exists to free researchers of politics, groupthink, fear of retaliation, and other issues that prevent “normal” employees from speaking truth to power. Researchers need to start using this privilege: They should speak up against questionable research and sloppy researchers, or at a bare minimum defend and protect the (often young) scholars who dare doing it.
Conclusion
Why did I decide to pursue this case? People often think that those who criticize other people’s work do it out of spite, jealousy, or revenge; that they are seeking clout, or that they have a personal agenda; that they are not good enough to do “real research” themselves, and so instead attack other people’s papers. The reason, at least for me, is much simpler. I was doing a PhD because I wanted to do science, and doing science doesn’t just mean building new things: It also means maintaining and cleaning what’s around you. This “janitorial work” is tedious and often thankless, but it is an integral part of our duties as scientists.
I am very proud of what I have accomplished. Despite the bullying, threats, and intimidation that characterized my PhD experience; despite being born and raised in a social environment that was far from being conducive to any form of accomplishment, I have contributed to something that has the potential to change our fields for the better. If academics have the courage to learn from what happened, if they have the collective intelligence to seize the moment and make necessary reforms, then we might look back on these scandals as pivotal moments in the history of our fields.
My concluding words will be for my fellow reform-minded behavioral researchers who, unlike me, have decided to stay in academia. First, know that you are not alone. Academia as a system is not necessarily rewarding truth-seeking right now, but the incredible success of the GoFundMe for Data Colada, and the hundreds of messages of support I have received since the New Yorker piece came out, suggest that there are many people out there who are unhappy with the current state of the field. Find them, and make sure you support each other: Strength is in numbers.
Second, you don’t need to be Data Colada to curb the negative impact of fraud and p-hacking: All you need to do is use your voice. If you have concerns about a paper, speak up. If you fail to replicate an effect, speak up. If you detect anomalies in a paper, speak up. If you think a journal or a professional society is making fraud easier to commit and harder to detect, speak up. Remind yourself that you haven’t done a Ph.D. (and endured the sacrifices that go with it) to stay silent, submit to bullies, and please people who have little regard for the scientific endeavor.
When the emperor has no clothes, you can choose to be the person blurting out the uncomfortable truth.